Category: PhD Research
Python for Simulations and Modeling in PhD Research: Advantages and Applications
23/05/2023
Simulations and modeling are essential tools in PhD research, enabling researchers to investigate complex phenomena, predict outcomes, and gain valuable insights. Python has emerged as a preferred language for conducting simulations and modeling in diverse fields of study. Its versatility, extensive libraries, and ease of use have made it a powerful tool for researchers seeking to simulate and model intricate systems. This blog will explore the advantages and applications of using Python for simulations and modeling in PhD research.
Furthermore, the availability of Python implementation service providers can assist researchers in streamlining their projects, providing expertise in Python programming, and ensuring efficient and effective implementation of simulations and models. Python implementation service offers valuable assistance to researchers, ensuring that their simulations and modeling efforts are optimized and deliver accurate results.
Application
Examples of Python for Simulations and Modeling in PhD Research:
Physics and Engineering: Python is widely used in simulating physical systems, such as quantum mechanics, fluid dynamics, and electromagnetism. Researchers can employ Python libraries like SciPy, NumPy, and SymPy to solve differential equations, perform numerical simulations, and analyze experimental data. For example, Python can be utilised to simulate the behavior of particles in particle physics experiments or model the flow of fluids in engineering applications.
Biology and Computational Biology: Python finds extensive applications in modeling biological systems and conducting computational biology research. Researchers can leverage Python libraries like Biopython and NetworkX to simulate biological processes, analyze genetic data, construct gene regulatory networks, and perform protein structure predictions. Python’s flexibility and ease of integration with other scientific libraries make it ideal for complex biological simulations.
Prior research
Title: “DeepFace: Closing the Gap to Human-Level Performance in Face Verification”
Summary: This research paper introduced DeepFace, a deep-learning framework for face verification. Python was used to implement the neural network models and train them on large-scale datasets.
Title: “TensorFlow: Large-Scale Machine Learning on Heterogeneous Distributed Systems”
Summary: This paper presented TensorFlow, a popular open-source machine learning framework. Python was used as the primary programming language for building and deploying machine learning models using TensorFlow.
Title: “PyTorch: An Imperative Style, High-Performance Deep Learning Library”
Summary: This research introduced PyTorch, another widely used deep learning library. Python was the primary language for implementing PyTorch’s computational graph framework and training deep neural networks.
Title: “Natural Language Processing with Python”
Summary: This research book focused on natural language processing (NLP) techniques using Python. It explored various NLP tasks, such as tokenization, part-of-speech tagging, and sentiment analysis, and implemented them using Python libraries like NLTK and spaCy.
Title: “NetworkX: High-Productivity Software for Complex Networks”
Summary: This research paper introduced NetworkX, a Python library for studying complex networks. It provided tools for creating, manipulating, and analyzing network structures, enabling researchers to explore network science and graph theory.
Title: “SciPy: Open Source Scientific Tools for Python”
Summary: This paper described SciPy, a scientific computing library for Python. It covered a wide range of topics, including numerical integration, optimization, signal processing, and linear algebra, all implemented in Python.
Advantages
Python offers several advantages for simulations and modeling in PhD research. Here are some key advantages:
Versatility and Rich Ecosystem: Python is a highly versatile programming language with a vast ecosystem of libraries and frameworks specifically designed for scientific computing, simulations, and modeling. Libraries such as NumPy, SciPy, Pandas, and Matplotlib provide robust tools for numerical computations, optimization, data manipulation, and visualization, making Python a powerful choice for PhD research.
Ease of Use and Readability: Python has a clean and readable syntax, making it easier to write, understand, and maintain code. It’s simplicity and high-level nature allow researchers to focus more on the problem at hand rather than getting lost in complex programming details. Python’s readability also enhances collaboration, as it is easier for others to review and understand the code, facilitating reproducibility and sharing of research findings.
Open-Source Community and Support: Python has a large and active open-source community, which means that researchers have access to a wide range of resources, forums, and documentation. This community actively develops and maintains numerous scientific libraries and packages, providing continuous improvements, bug fixes, and new features. It also enables researchers to seek help, share code, and collaborate with experts in their respective domains.
Integration with Other Languages and Tools: Python’s flexibility extends to its ability to integrate with other languages and tools. Researchers can easily combine Python with lower-level languages like C and Fortran to leverage their performance benefits. Additionally, Python can be seamlessly integrated with popular simulation and modeling software, such as MATLAB, allowing researchers to leverage existing tools and codes while benefiting from Python’s versatility.
Reproducibility and Replicability: Python’s emphasis on code readability and documentation contribute to the reproducibility and replicability of research. By using Python for simulations and modeling, researchers can write clean, well-documented code that can be easily understood and reproduced by others. This fosters transparency in research and allows for independent verification and validation of results.
Computational Efficiency: While Python is an interpreted language, it offers several tools and techniques to optimize performance. Libraries like NumPy and SciPy leverage efficient algorithms and data structures, while packages such as Numba and Cython enable just-in-time (JIT) compilation and speed up computationally intensive parts of the code. Additionally, Python provides interfaces to utilize multi-core processors and distributed computing frameworks, allowing researchers to scale their simulations and models to large-scale systems.
Verification, validation, and uncertainty quantification of Python-based simulations and modelling
Verifying, validating, and quantifying uncertainties in Python-based simulations and modelling for PhD research involves a comprehensive process to ensure the reliability, accuracy, and credibility of your simulations and models. Here is a general framework to guide you through the steps:
Define verification, validation, and uncertainty quantification (VVUQ) objectives: Clearly articulate the specific objectives you want to achieve in terms of verification, validation, and uncertainty quantification. Determine the metrics and criteria you will use to assess the performance and reliability of your simulations and models.
Develop a verification plan: Establish a plan for verifying the correctness and accuracy of your Python-based simulations and models. This may involve activities such as code inspection, unit testing, and comparison against analytical solutions or benchmark cases. Define the specific tests and criteria you will use to verify different aspects of your simulations and models.
Conduct code verification: Perform code verification to ensure that your Python code accurately implements the mathematical and computational algorithms of your simulations and models. This can involve using analytical solutions or simplifying cases with known results to compare against the output of your code.
Plan the validation process: Develop a validation plan to assess the accuracy and reliability of your simulations and models by comparing their results against experimental data, empirical observations, or field measurements. Define the validation metrics, experimental setup, and data requirements for the validation process.
Gather validation data: Collect or obtain relevant experimental data or observations that can be used for the validation of your simulations and models. Ensure that the validation data covers the same phenomena or scenarios as your simulations and models and is representative of real-world conditions.
Perform model validation: Run your Python-based simulations and models using the validation data and compare the results with the corresponding experimental data. Assess the agreement between the simulation results and the validation data using appropriate statistical measures and visualization techniques. Analyze and interpret any discrepancies or deviations and identify potential sources of error or uncertainty.
Quantify uncertainties: Identify the sources of uncertainties in your simulations and models, such as input parameter variability, measurement errors, or modeling assumptions. Implement techniques for uncertainty quantification, such as Monte Carlo simulations, sensitivity analysis, or Bayesian inference, to characterize and propagate uncertainties through your simulations and models.
Validate uncertainty quantification: Validate the uncertainty quantification process by comparing the estimated uncertainties against independent measurements or other sources of information. Assess the reliability and accuracy of your uncertainty estimates and refine them if necessary.
Document and report: Document your verification, validation, and uncertainty quantification processes, methodologies, and findings. Clearly describe the steps taken, the results obtained, and the conclusions drawn from the VVUQ analysis. Communicate your findings through research papers, reports, or presentations to demonstrate the credibility and robustness of your Python-based simulations and models.
Continuously update and improve: VVUQ is an iterative process, and as new data, insights, or techniques become available, it is important to continuously update and improve your simulations and models. Incorporate feedback, learn from validation exercises, and refine your simulations and models to enhance their accuracy, reliability, and usefulness for your PhD research.
Why Is Python Good For Research? Benefits of the Programming Language
Python is widely recognized as a popular programming language for research across various fields. Here are several reasons why Python is considered beneficial for research:
Ease of use and readability: Python has a clean and intuitive syntax, making it easy to learn and use, even for individuals with minimal programming experience. Its readability and simplicity enable researchers to quickly prototype ideas, experiment with algorithms, and focus on the research problem rather than struggling with complex programming concepts.
Extensive scientific libraries: Python offers a rich ecosystem of scientific libraries and frameworks that cater specifically to research needs. Libraries such as NumPy, SciPy, pandas, and scikit-learn provide powerful tools for numerical computing, data analysis, machine learning, and statistical modeling. These libraries streamline research tasks, eliminate the need to reinvent the wheel and enable researchers to leverage existing optimized functions and algorithms.
In conclusion, Python has proven to be a valuable asset in simulations and modeling for PhD research, offering a wide range of advantages and applications. Its ease of use, extensive scientific libraries, interoperability, and supportive community make it an excellent choice for researchers seeking to develop accurate and efficient simulations. Python’s versatility allows for seamless integration with other languages and tools, further enhancing its capabilities in interdisciplinary research. Moreover, the availability of Python implementation service providers can significantly support researchers, providing expertise and assistance in implementing simulations and models. With Python’s robust ecosystem and collaborative nature, researchers can explore new frontiers, tackle complex problems, and contribute to advancements in their respective fields through simulations and modeling.
If you want our help in this regard, then you can visit our website https://www.fivevidya.com/python-projects.php to learn more.
Thank you for reading this blog.
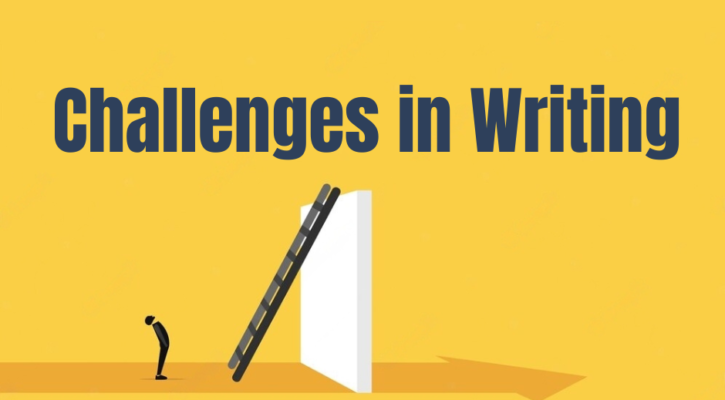
The Challenges in Writing a Multi Authored Academic Publication, More Co-authors for your research paper.
03/04/2023
Co authorship is when two or more authors collaborate to publish a research article. There has been a significant increase in multi disciplinary research which has also expanded multi authored academic research in the past few years. There are a variety of reasons for this growth, most commonly researchers attribute this growth to the incremented pressure of publication for career growth and promotions. With such a requirement, co authorship is often a lucrative return on investment. Another reason because of which collaborative writing
has increased are, better opportunities for co authorship as networks have increased and advances in technological developments have made it very convenient to work on the same text electronically.
Multiple authorship can be useful for the authors in various ways but it comes with its own set of challenges and conflicts. The various issues that can flare up in multi authored research need to be addressed so as to pre warn the authors to avert possibility of disputes.
There are few questions that arise upfront the moment we talk of co authorship in research.
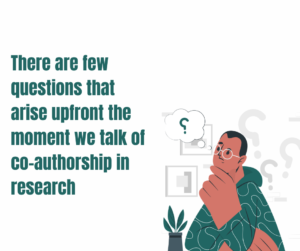
Amongst the first few ones are:
a) What should be the order of names in the paper? The names should be listed on the criterion of contribution in the paper, seniority, alphabetically or any other logic should be applied to decide the first position in the names of the authors?
b) How to correctly justify the contribution of an academic colleague to grant him authorship credit. The question becomes more explicit. When we are talking in context of a PhD supervisor or research grant holder whose name sometimes is added merely by the virtue of their position.
These questions cannot be ignored as the credits of an author are a very crucial criterion for professional growth, getting funding and at the same time it is an important concern of publishing ethics.
Challenges associated with Co-Authorship
Paucity of appropriate training and guidance
Most of the researchers do not receive any training on co authorship and at the same time they also feel that the institution does not offer any special support for any kind of collaborative writing.
Confusion and Dissatisfaction:
Many researchers do not have enough clarity about this practice of co authorship and its resulting implications. Moreover, the increasing concept of co authorship has increased the intervention of senior professors and project guides in getting their names attached when a
paper comes to them for review. Senior academicians often get over credited and the junior ranked scholars and academicians do not get their dues for the amount of effort they put in. This leads to a general trend of dissatisfaction and confusion amongst the budding
researchers to emphasise towards co authorship.
Lack of Co authorship policies:
Institutions do not have co authorship policies and ethics placed in a structured manner which can help researchers to overcome the issues that overwhelm them in this regard. Institutions need to create a framework for co authorship policies and disseminate them amongst the
fraternity so that the best practices in the field are encouraged.
Acceptance of Diversity of work styles:
Not all researchers can have the same work style and when the author are three or more, clashes in work styles are imperative. It requires a whole lot of adjustment to get along with the working styles of other researchers. Most of the time this adjustment is accepted to be
from the end of the junior researchers where the senior professors and research guides don’t show any flexibility in their working styles with other budding researchers.
Miscommunication amongst the authors
The English saying, “ Too many cooks spoil the broth” applies pretty well in the concept of co-authored research tools. Clashes and miscommunication on the grounds of various factors is bound to create friction amongst the authors. All the details and approach should be
decided well in advance before starting to write the paper. This can surely minimise the complications that can arise because of miscommunication, but still there is always scope for friction in some form or the other Inappropriate writing tools or approaches:
Not all authors who choose to write under collaboration can have the same command over research tools or even gel into having the same approach towards writing. This can disrupt the uniformity of the paper in terms of the quality as well as the style for research. It not only affects the writing quality but many a times even frustrates the authors when they feel that the contribution of the other author is affecting the outcome of the research.
10 Commandments of Co-Authorship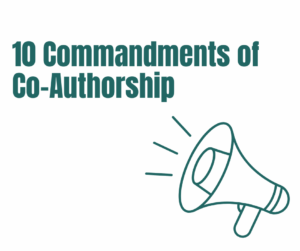
A multi authored paper can be a outcome of a common discrete research project or even at times a much larger project that incorporates different papers based on common data or methods. It calls for a meticulous planning and collaboration amongst team members. The commandments of co authorship brought forth here can be applicable at the planning as well as the writing stage of the paper. The authors can come back to the rules from time to time for reiteration and it can surely build a foundation of a constructive research.
Commandment 1:
Contemplate well in choosing your co-authors The writing team is formulated at the formative stage of writing the paper. The pre requisite for choosing co-authors should be expertise and the interest area of research of the co- authors. This can be done by understanding the expectations of all the contributors in research and set up a research goal and also see that the researchers are up to working collectively towards the common goal. This step needs to be headed by the project leader who needs to identify the varied expertise required for making the paper. And also define the criterion that can be applied for qualifying as a co-author. There can be a situation of doubt in selecting co-authors sometimes and being inclusive in approach here does the trick. The roles
and responsibilities of the different authors can also be broadly defined at this stage.
Commandment 2:
Proactive Leadership strategy
A proactive leader is critical for a multi-authored paper so that the outcome is satisfactory in nature. The leader, who most of the time is the first author in the research paper should follow the approach of consensus building and not hierarchical approach while parallelly
working on the manuscript structure and keeping an overall vision of the paper alive.
Commandment 3:
An effective Data Management Plan(DMP)
A DMP that has been created and circulated at the initial level of the paper creation should have the approval of all co-authors. The DMP is a broad outline stating the way in which the data will be shared, versioned, stored and curated and the access to the data will be given to which all authors at different stages of the research project. The DMP should be a simple document but detailed and should be summarized in a couple ofparagraphs. All the data providers and co-authors should agree with the DMP confirming that it has the consensus of the institutional requirements as well as the funding agency.
Commandment 4:
Clearly define the authorship prerequisites and guidelines
Transparent authorship guidelines help to avert confusions and misunderstanding at all stages of the research. Depending on the amount of contribution by the author, the author order can be created with the person who contributed the most being right in the front. In situations where all authors have contributed equally alphabetical order can be used to create the order. At times some contributors might be there who do not meet the author expectations and predefined conditions. The alternative for them is to be included in the list of contributors in acknowledgement. The author order must be revisited and re considered during the process when the roles and responsibilities keep changing.
Commandment 5:
Give framework of writing strategy
He writing strategy that you decide for your co-authored article should be adopted according to the needs of the team. There can be one principle writer for the paper if the data is wide ranging in nature. The most commonly used strategy is when the paper is split into separate sub sections and the different authors are held responsible for sections based on their individual expertise and interest. Whatever may be the strategy it should. Be all inclusive of the co-authors, engaging them from the narrative stage to final structuring of the paper. Thiss urely helps in case the need arises to rewrite any part of the paper at any given later stage.
Commandment 6:
Choosing Appropriate Digital Tools
If you choose to do interactive writing for your paper, you may need aid of such platforms that support synchronous work as the whole group is writing together such as Google Docs On the contrary, those papers that are written sequentially or the principle author is doing the
writing task predominantly conventional platforms such as Ms Word may also work. At the preliminary stage itself , plan should be created for comments and tracking changes. In addition to the tool for writing, you will have to choose a platform for virtual meetings from time to time. You will need to compare the platforms on specific requirements you might have for your research meetings such as number of participants possible in a single group call, permission to record and save the meetings, screen casting or note making authority. With multiple options available in the present time for virtual interactions, customised options are there at a cost effective manner making it easier to choose.
Commandment 7:
Defining clear realistic and rigid timelines
Deadlines help to maintain the momentum of the group and facilitates on time completion of the paper. When you are a co-author, you must look at the deadlines se by the group leader from your on perspective and see that with all your other commitments will you be able to
live by them. Respect other authors time as well by being on time for meetings, schedules. By developing a culture of positivity and encouragement in the team keeping deadlines becomes easier and staying on task can be accomplished. Always remember that collaborative writing needs more revisions and reading sessions as compared to solo research papers.
Commandment 8:
Communicate effectively with all co-authors
Being transparent and explicit about expectations and deadlines helps to avert misunderstandings in the mind of the authors and helps to keep conflicts at bay. If you are the project leader make all consequences clear to the authors, like when they do not meet deadlines or if someone breaks the group rules. Consequences could vary from change in the authors order or removing authorship. If one co-author does not work well in the team, possible chances are he would not be included in the same team in the forthcoming research.
Commandment 9:
Collaborate with authors from diverse background and design participatory group model
When there are multiple authors in a research , chances are there that they may belong to different cultural or demographic backgrounds. It surely brings in a wider perspective to research and beings inputs from different backgrounds. There may be some language barriers
and they need to be overcome by a more empathetic approach towards the non-native speaker authors to allow them to express their opinions. A well curated participatory group model can be used to crate platforms for opportunity of expression for different personalities in
authorship.
Commandment 10:
Own the responsibility of co authorship
There are benefits in being a co-author and at the same time it comes with responsibilities. You have to owe responsibility of the paper as a whole and that you have checked the accuracy of the paper as a whole. One of the final steps begore submitting a co-authored paper are that all authors have given their consensus on their contribution and approval of the final outcome of the paper and also support its submission for publication finally.
Finally…
Despite a lot of challenges and obstacles in writing multi authored papers that have been discussed above, co-authored papers are the trend and they come with their own set of benefits that cannot be denied. There is no thumb rule in handling the challenges but the above commandments can be read and reread to customise according to the requirements of your team and its vision…If you still have never attempted a co-authored paper, the time is now. Go by the trend in research.
Knowledge, Research Questions and Research Paradigm
29/01/2021
The research question thus posed the objective for exploring and discovering processes that led to knowledge creation. Knowledge by itself is a difficult construct which is viewed from various disciplinary perspectives such as philosophy, cognitive psychology, sociology can pose a myriad and often conflicting variety of definitions. Broadly there are western philosophies which posit separations of reality of physical word from the individual while there are eastern that stress upon knowledge to be occurring to the whole personality and character rather than in separated components of body and mind.
According to the interpretive approach knowledge is personal and constructed through interpretations of meanings. Knowledge is emergent and path dependent. Therefore, by building a linked structure of event pathways the process of accumulation of knowledge and its creation can be constructed. Such study involved collection of naturalistic data and observations and interpretation and validation of meanings. It required creation of a framework for undertaking the research.
The interpretive research paradigm however is relatively new with its traditions yet to be fully established. Only a few works existed to demonstrate the use of methods for data collection analysis and theory building. Apart from principles suggested by Lincoln and Guba (1985) and Klien and Myers (1999) which were yet to be widely applied and agreed upon there were no standards for conduct and evaluation of such research.
Why Pilot Questionnaires? Reliability and Validity Testing for PhD Research
27/03/2020
There are two keys tests for a questionnaire: reliability and validity. A questionnaire is reliable if it provides a consistent distribution of responses from the same survey universe. The validity of the questionnaire is whether or not it is measuring what we want it to measure
Testing a questionnaire directly for reliability is very difficult. It can be administered twice to the same of test respondents to determine whether or not they give consistent correct answers.However,the time between the two interviews cannot usually be very long ,both because the respondent’s answer may in fact change over time and because, to be of value to the researcher, the results are usually required fairly quickly. The short period causes further problems in that respondents may have learnt from the first interview and as a result may alter their responses in the second one .Conversely, they may realize that they are being asked the same questions deliberately try to be consistent with their answers. In testing for reliability, we are therefore often asking whether respondents understand the questions and can answer them meaningfully.
Testing a questionnaire for validity requires that we ask whether the questions posed adequately address the objectives of the study. This should include whether or not the manner in which answers are recorded is appropriate.
In addition, questionnaires should be tested to ensure that there are no errors in them. With time scales to produce questionnaires sometimes very tight, there is very often a real danger of errors.
Piloting questionnaires can be thus divided into three areas: reliability., validity, and error testing.
Reliability
- Do the questions sound right? It is surprising how often a question looks acceptable when written on piece of a paper but sounds false, stilted or simply silly when read out.it can be salutary experience for questionnaires elves writers to conduct the interview themselves .They should note how often they want to paraphrase a question that they have written to sound more natural,.
- Do the interviewers understand the questions? Complicated wording in a question can make it incomprehensible even to the interviewers. If they cannot understand it there is a little chance that respondents will.
- Do the respondents understand a question? It is easy for technical technology and jargon to creep into questions, so we need to ensure that it is eliminated.
- Have we included any ambiguous questions, double barreled questions, loaded or leading questions?
- Does the Interview retain the attention and interest of respondents throughout? If attention is lost before it wavers, then the quality of the data may be in doubt. Changes may be required in order to retain the respondent’s interest.
- Can the interviewers or respondents understand the routing instructions in the questionnaire? Particularly with paper questionnaire, we should check the routing instructions can be understood by the interviewers, or if completion, by respondents
- Does the interview flow properly? The questionnaire should be conducting a conversation with the respondent. A questionnaire that unfolds in a logical sequence, with a minimum of jumps between apparently unrelated topics, helps to achieve that.
Validity
- Can respondents answer the questions? We must ensure that we should ask the questions that they are capable of providing answers.
- Are response codes provided sufficient? Missing response codes can lead to answers being forced to fit into the codes provided, or to large numbers of ‘other’ answers.
- Do the response codes provide the sufficient discrimination? If most respondents give the same answer, then the pre-codes provided may need to be reviewed to see how the discrimination can be improved, and if that cannot be achieved, queries should be raised regarding the value of including the question.
- Do questions and responses answer the brief? We should by this time reasonably be certain that the questions we think we are asking meet the brief ,but we need to ensure that the answers which respondents give to those questions are the responses to the questions that we think we are asking.
Error Testing
- Have mistakes been made? Despite all the procedures that most research companies have in place to check questionnaire before they go live, mistakes do occasionally still they get through. It is often the small mistakes that go unnoticed, but these may have a dramatic effect on the meaning of a question or on routing between questions. Imagine the effect of inadvertently omitting the word ‘not’ from a question.
- Does the routing work? Although this should have been comprehensively checked, illogical routing sequences sometimes only become apparent with live interviews.
- Does the technology work? If unusual or untried technology is being used perhaps as an interactive element or for displaying prompts this should be checked in the field. It may work perfectly well in the office, but fields conditions are sometimes different, and a hiatus in the interview caused by slow working or malfunctioning technology can lose respondents.
How long does the interview take? Most Surveys will be budgeted for the interview to take a certain length of time .The number of interviewers allocated to the project will be calculated partly on the length of the interview ,and they will be paid accordingly .Assumptions will also have been made about respondent cooperation based on time taken to complete the interview .The study can run into serious timing and budgetary difficulties , and maybe impossible to complete if the interview is longer than time allowed for. Being shorter than allowed for. Being shorter than allowed for does not usually present such problems but may lead to wasteful use of interviewer resources.